Setting Reasonable Expectations for the Use of AI in Manufacturing Supply Chains: A Discussion with Attorney Peter Loh
Peter talks about AI projects in manufacturing supply chains to effectively forecasting market demands, procuring proper volume of materials and supplies, managing budget planning and more.
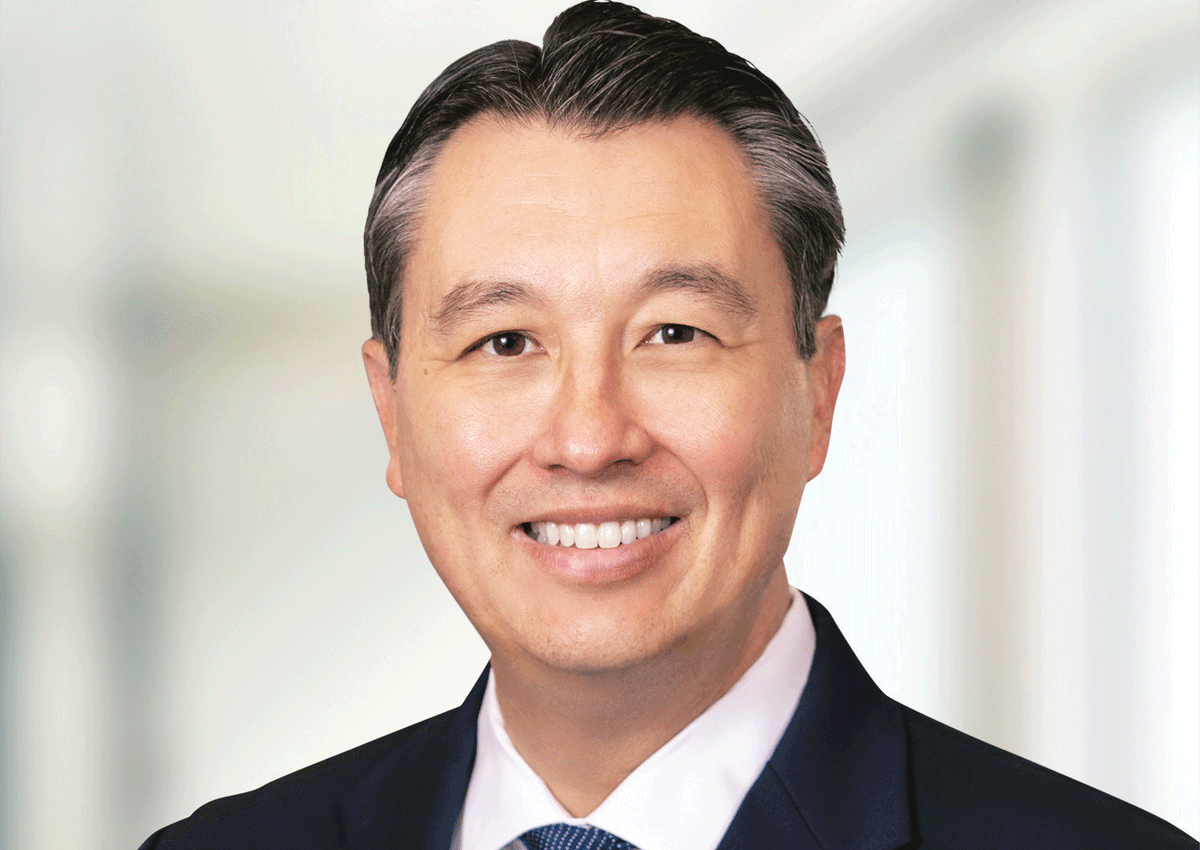
Can artificial intelligence (AI) meet vendor claims and produce noteworthy efficiencies and cost-reductions in manufacturing supply chains? What can manufacturers do to ensure that their AI projects will succeed? I’ve written in the past about AI implementations falling short of expectations, yet I remain positive about the technology’s future impact as algorithms and models become more sophisticated and refined.
I recently spoke with litigation attorney Peter Loh, who is a partner at Foley & Lardner. Peter works on client claims involving failed software implementations in manufacturing environments and is vice chair of the firm’s distribution and franchise team. We talked about some of the challenges manufacturers face in using AI, such as effectively forecasting market demands, procuring the proper volume of materials and supplies, and managing budget planning and logistics and distribution.
Mark: How has artificial intelligence changed the way manufacturers forecast demand?
Peter: Well, quite a few ways. One way would be enhanced accuracy. AI, using machine learning algorithms, can analyze large datasets instantaneously to identify patterns and trends that other traditional methods and humans might miss. And the models can improve over time as they learn from new data, leading to more accurate forecasts. You're, of course, taking out human error and subjective judgment, or emotion, that can play into forecasting in a traditional sense. You also have the ability for continuous updates based upon all the data that could be coming from various sources that the humans just couldn't – don't have the capacity to – absorb: sales data, market trends, economic indicators, even social media…. AI absorbs all that, processes it, synthesizes it and, again, comes up with some kind of conclusion. And [it] can adjust on-the-fly.
Mark: In manufacturing operations, specifically, what are some ways AI has improved procurement?
Peter: Demand-forecasting is going to [get] help here, because if you get more accurate forecasts, then you're going to be able to adjust procurement accordingly, optimizing inventory levels and reducing the risk of overstocking. You can also use AI to assess supplier performance based upon delivery times, quality of goods, and costs to ensure you're choosing the best suppliers. That can also help maintain relationships, because you are streamlining things and automating more. I think counterparties and suppliers may appreciate that increased efficiency.
In procurement, AI can also help with the cost optimization – negotiating better prices, optimizing purchase-order quantities, detecting different trends and abilities to predict future price movements. You're also going to be able to automate things, such as order processing, invoicing, contracts-management – the things that humans would have to do. AI can also help with risk management, forecasting things like natural disasters or financial instability, [and] market shifts.
Mark: Looking at budget-planning and capital expenditure and related projects, how have you seen AI help manufacturers improve their budget planning?
Peter: I think it's a lot of the things that we've already talked about, right? This increased ability with AI to absorb and synthesize mass amounts of data very quickly and learn different trends will certainly help with budgeting and planning for capex (capital expenditure) projects.
If you can predict costs and shifts in market demand, then you're going to be able to plan for your capex projects more efficiently and more accurately – from materials for labor, to equipment, to related expenses. And then, you're going to be able to roll in this real-time market data you are getting to help with understanding what the market is doing, including forecasting future demand to help you make those decisions. AI might also help with understanding risks associated with capex projects, analyzing stored data on similar projects under similar market conditions – better understanding [of] how and why did those capex projects pan out. How did they help the business or were they detrimental? AI could run through different scenarios based upon various models or various input factors and then provide some kind of forecasted results [that] executive management could compare against. Basically, I think AI is going to help with the decision-making process. AI is going to give executive management teams so much more insight on a macro level to make these decisions on the micro level.
Mark: What ways have you seen AI being implemented to improve manufacturing logistics and distribution? For example, where suppliers are pipelining raw materials – or sub-assemblies from sub-contract manufacturers – into the factory or contract manufacturer’s facility, or where finished goods are transported to resellers and distribution hubs?
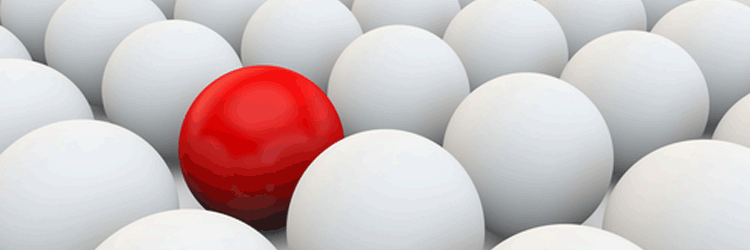
Peter: So, here, you're looking at just vastly increased efficiency based upon analyzing things as mundane as traffic conditions, fuel costs, travel time, [and] other operational costs that are associated with getting a point-A-to-point-B picture to look at – again, greater efficiency. You can then adjust delivery schedules or pickup times accordingly for, again, factoring in traffic, weather, other things that are going on in the demand-profile that could affect those things. AI is ensuring or helping to ensure timeliness, and … matching up inventory levels to demand so that you are not over-stocked or under-stocked. And then [it’s] automating the reordering process and being able to predict when your customers are going to want things again, probably based upon cyclical demand, season or weather, or other things that are going on in the market.
There is also warehouse or storage facility-level work, down to the granular level, where things are placed in a warehouse to maximize efficiency or minimize holding times for packing, and picking up, and storage … moving things around to make sure your warehouse operations are moving as smoothly as possible. You could also get into blockchain technology to ensure transparency and traceability of goods moving from point A to point B to point C to point D, optimizing allocation of the inventory across multiple distribution centers based upon [a] demand pattern. Should I have this widget in Toledo, or should I store this widget in Jacksonville, Florida? Again, [this can be] based upon whether traffic, other logistical hurdles, the location of my customers, and when they're going to want things, versus when other customers are going to want things.
Manufacturers could also coordinate and collaborate and share some of this data with your suppliers so that they understand what you're doing, because a lot of this is kind of a dance, right? And so it helps that the dance partner knows what you're doing before you're doing it so that he can mirror or match his activities to yours. All this, of course, will help lower costs, because if you're more efficient with things like fuel and other inputs that you're having to purchase, this will bring down costs. It will also bring down labor. You don't want to have 50 drivers on the payroll if you only need 25. You don't want 100 warehouse workers if you only need 60.
Mark: For manufacturers you have worked with who have implemented AI, which departments or processes have you seen the greatest improvements in their performance metrics?
Peter: Reduced downtime. As far as machinery and equipment, reducing [the amount] of equipment [needed], or forecasting equipment-costing based on capacity forecast needs or accounting for equipment failures – these are some areas [where it has improved performance]. On the flipside, [it’s been helpful for] maintaining higher levels of production-line uptime and then optimizing production schedules, ensuring the efficient use of resources, and minimizing idle time with your labor. I think those are the big things.
Secondarily, [it] is also helping with quality control – the capability to identify defects with greater accuracy than human inspectors [can do] and … being able to analyze the processes used for identifying incorrect quality issues.
I think those two areas are the biggest. From what I'm hearing, people are looking for [and] hoping to achieve greater help in overall supply-chain management in the routing and scheduling of the transportation, delivery times and costs, and things like this.
Mark: What are some things manufacturers often forget to think about when establishing AI projects and creating AI project goals and objectives?
Peter: Machines will only give you the quality of the output depending on the quality of your input. We have to understand that this is kind of a gating issue. The data that's going in has to be accurate. It has to be as complete and as comprehensive as possible. This is axiomatic, or obvious.
The other challenge is integrating massive amounts of data from various sources. This required effort can often be underestimated. And then, the other question or challenge manufacturers need to address is, what are we doing? Why? Again, the machine is only going to do what we ask it to do. We have to have very clearly defined goals and objectives. What is it that we're hoping to accomplish? Manufacturers also have to ask, Do we have the necessary computing power, the data storage, the networking capabilities [to do this]? Do we have the infrastructure to run something like this?
Mark: Is there anything else we've not talked about that you feel readers should know?
Peter: Yes. This point doesn't go to manufacturing, but it relates to just what I've learned and [it] aligns with insights from other people who are active in this space. The potential amount of change and amount of advancement that we could see from AI – to say [this] would be exponential is probably a grave understatement.
AI is not going to affect just manufacturing. It will affect everyone's lives, period, and [it] will [do so at a] level [on par with] the discovery of fire or the invention of the wheel. The amount of impact AI will have on all of us socially, economically, culturally, I think, will be very eye-opening [in] the next five to ten years.